Meet our Students!
Maegan Tucker
Advisor: Aaron Ames // Mechanical Engineering // G4
Born to two engineers, Boston native Maegan Tucker's interest in engineering started at an early age. With an interest in problem solving and a passion for helping people, Maegan attended Georgia Tech where her research into above-the-knee prosthetics led her to pursue a PhD in Mechanical Engineering at Caltech. Maegan was drawn to Caltech's small size and collaboration-focused community. In the spirit of collaboration, Maegan recently co-wrote a paper with fellow classmate, Ellen Novoseller, that won best conference paper at the International Conference of Robotics and Automation. Her co-author added an important machine learning component to her studies, illustrating the power of cross-disciplinary collaboration that is facilitated by the intimate size of Caltech. Maegan's passion for mechanical engineering is evident even when she is not in the lab. She spends her free time designing and building mechanical keyboards from scratch. She prefers the feel of mechanical keyboards over mass-produced ones and enjoys how customizable they can be. She says "Everyone should give them a try!"
Maegan's research focuses on developing exoskeleton technology. Specifically, Maegan's interest is in the "science of walking." Focusing on theory, Maegan studies how humans walk and how robots can replicate this activity. Using mathematics, she characterizes the stability and robustness of walking robots, and hopes to develop robots with a natural and comfortable walking pattern that can assist people. The exoskeletons Maegan works with are not only programmed to physically aid their wearers, but also to learn from their muscle movements! Post-Caltech, Maegan will pursue further studies as a postdoctoral fellow and hopes to ultimately run her own lab. More about Maegan's work can be found at: https://breakthrough.caltech.edu/story/maegan-tucker-robotic-mobility-caltech-roam/
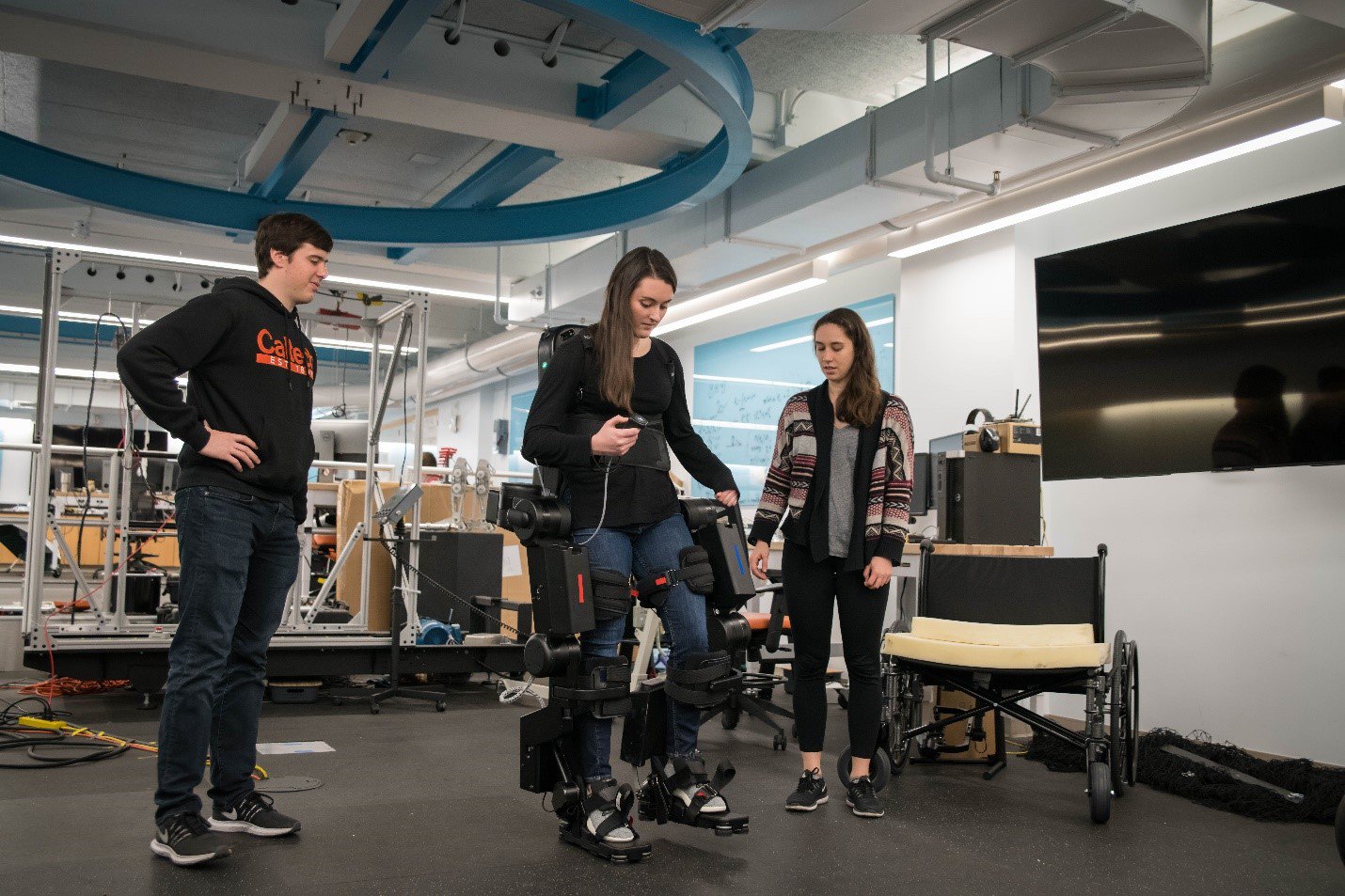
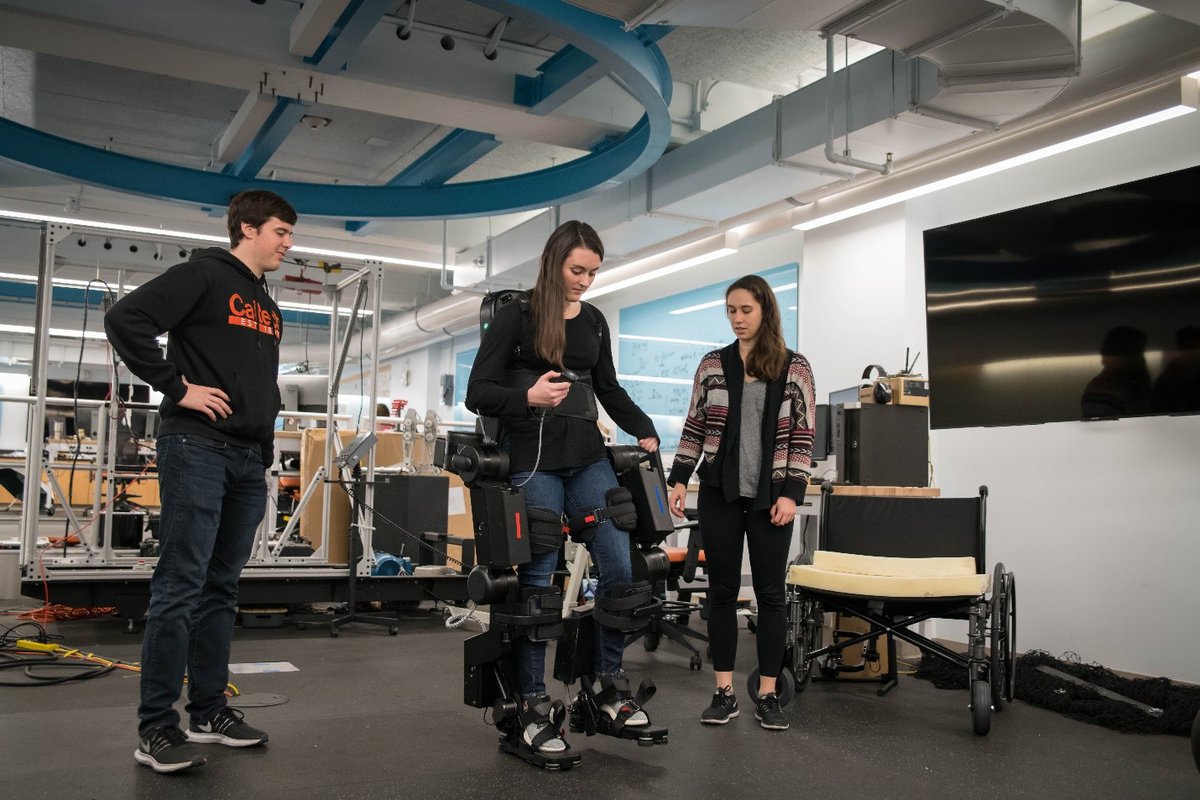
Charles Sanfiorenzo
Advisor: Kaihang Wang // Biology // G3
Born and raised in Puerto Rico, Charles Sanfiorenzo studied cell and molecular biology at the University of Puerto Rico. After interning at the Massachusetts Institute of Technology, Charles developed an interest in the regulation of messenger RNA and chose Caltech for graduate studies based on its strength in RNA biology. Charles is impressed with Caltech's tight-knit community of diverse and passionate students and has especially enjoyed working with the brilliant undergraduates here.
In Kaihang Wang's laboratory in the Division of Biology and Biological Engineering, Charles has devoted himself to developing powerful new tools to address questions in synthetic biology. Using bacteria as a model system, he is using bacterial conjugation to transfer large portions of the genome between different species of bacteria. Ultimately, this approach will allow him to explore how the genome determines the boundaries between distinct species of bacteria. He is also working to extend this technology into mammalian cells. Charles appreciates the lab's exploratory nature, which gives him the freedom to pursue innovative projects.
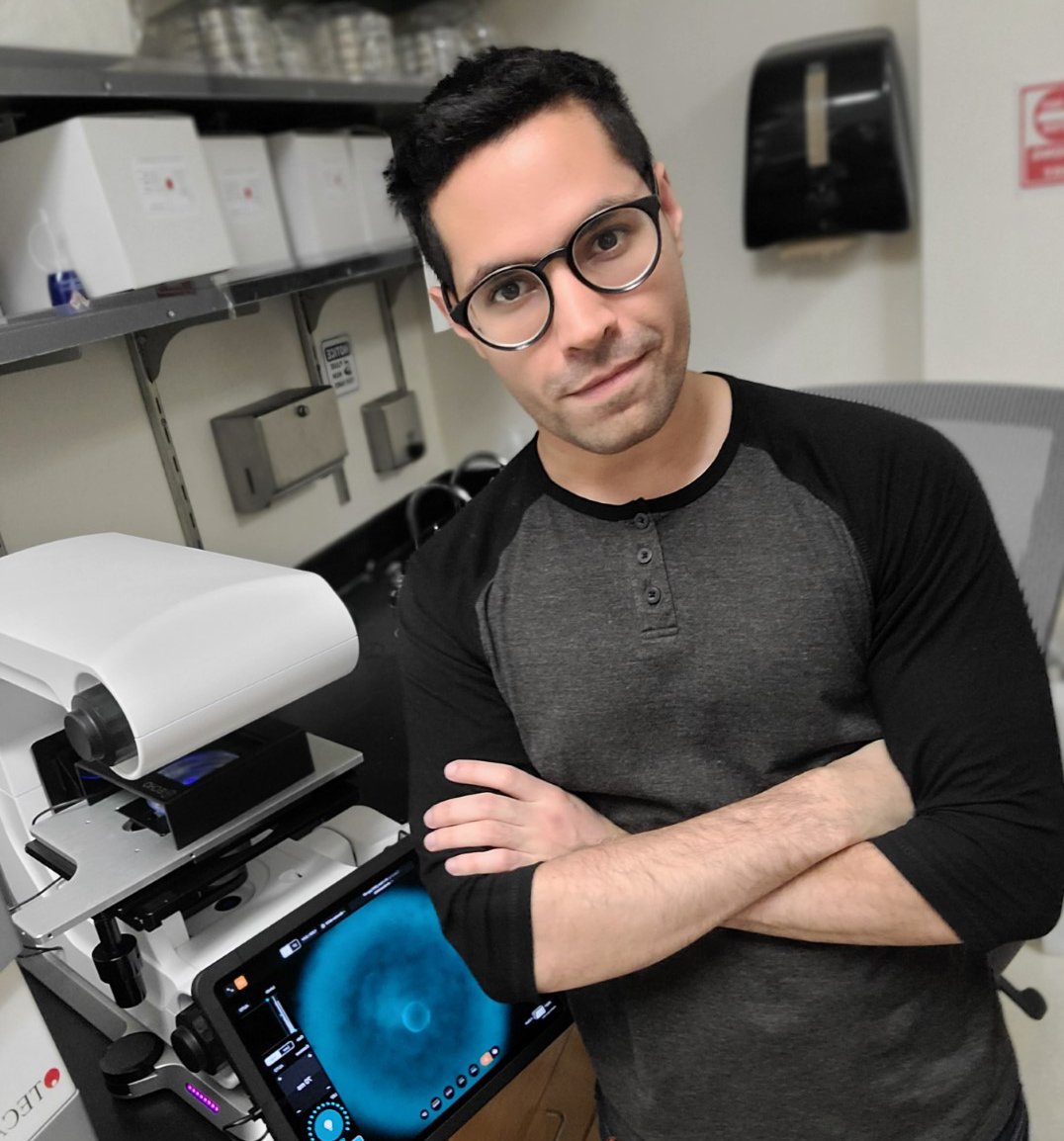
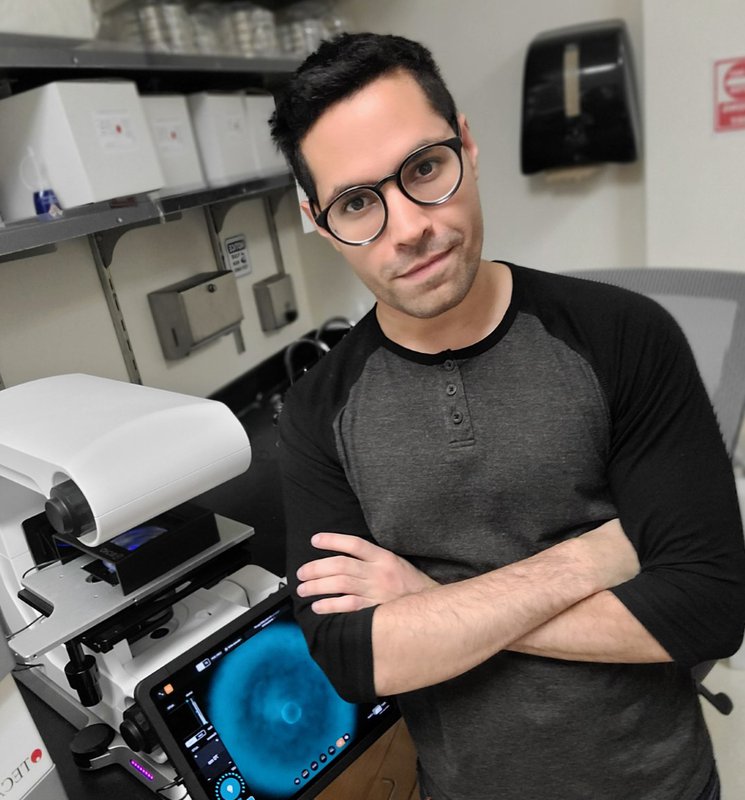
Zhuoran Qiao
Advisor: Thomas Miller // Chemistry // G2
Proud to call himself the first scientist in his family, Zhuoran Qiao has always been motivated by a desire to understand how the world works through science. After undergraduate study at Peking University and an internship at Harvard University, Zhuoran came to Caltech from China to pursue a PhD in Chemistry. Zhuoran feels that one of Caltech's greatest strengths is the passion of its students and faculty to achieve great discoveries that impact society as a whole.
Zhuoran arrived at Caltech with a passion for using artificial intelligence to explore the behavior of quantum mechanical systems, which is previously only accessible by solving the wave function from the Schrödinger equation. He has gone on to develop a novel strategy called "OrbNet" that uses machine learning to efficiently predict solutions to Schrödinger's equation, including its application to larger molecules like proteins. OrbNet is able to achieve accurate solutions with far cheaper computational cost compared to previous methods. Zhuoran's breakthrough has already made waves, being chosen as an Editor's Pick in the Journal of Chemical Physics. His method has many practical applications. For example, it can be used to predict binding affinities between a ligand and various drugs, and has been used by pharmaceutical companies like Amgen.
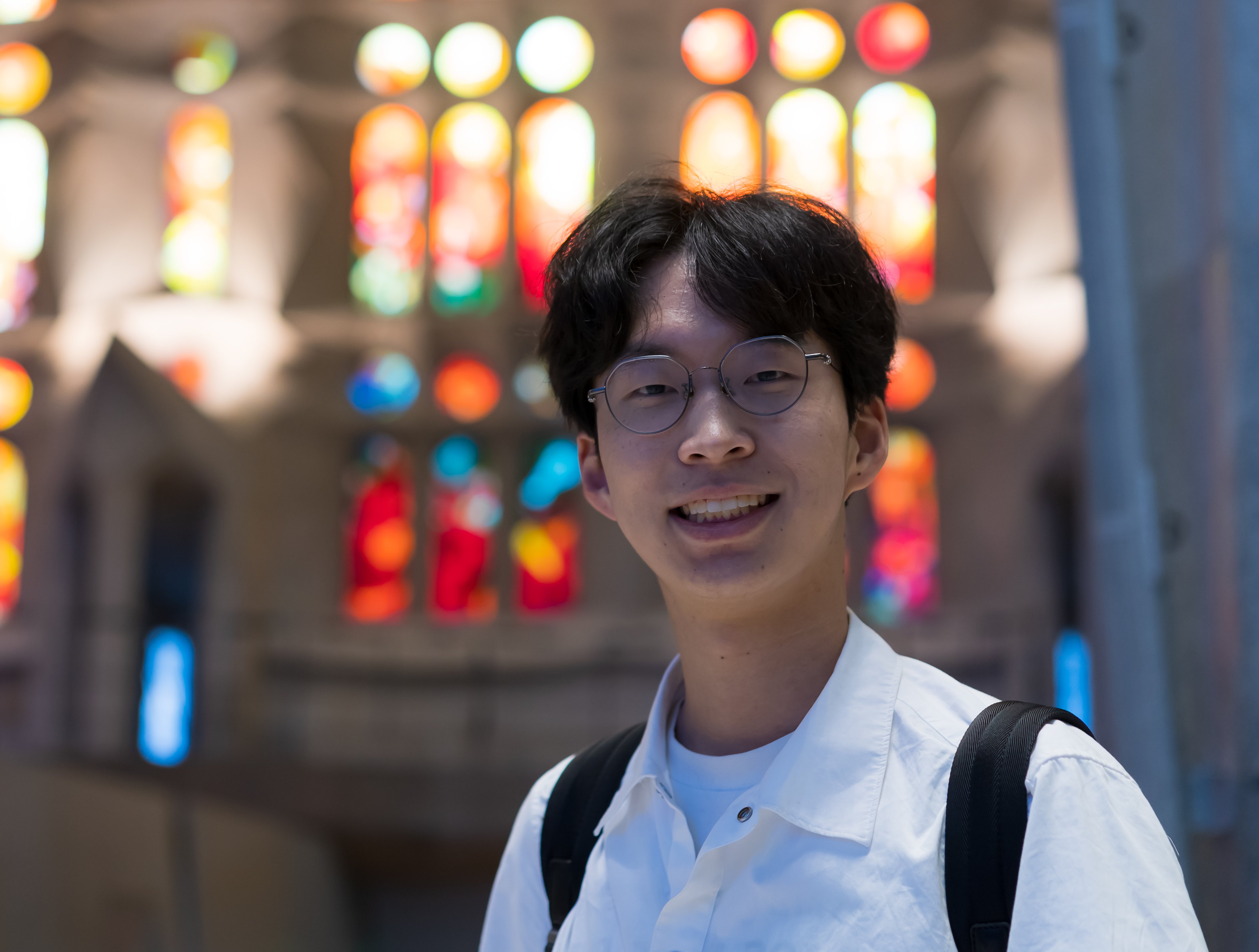
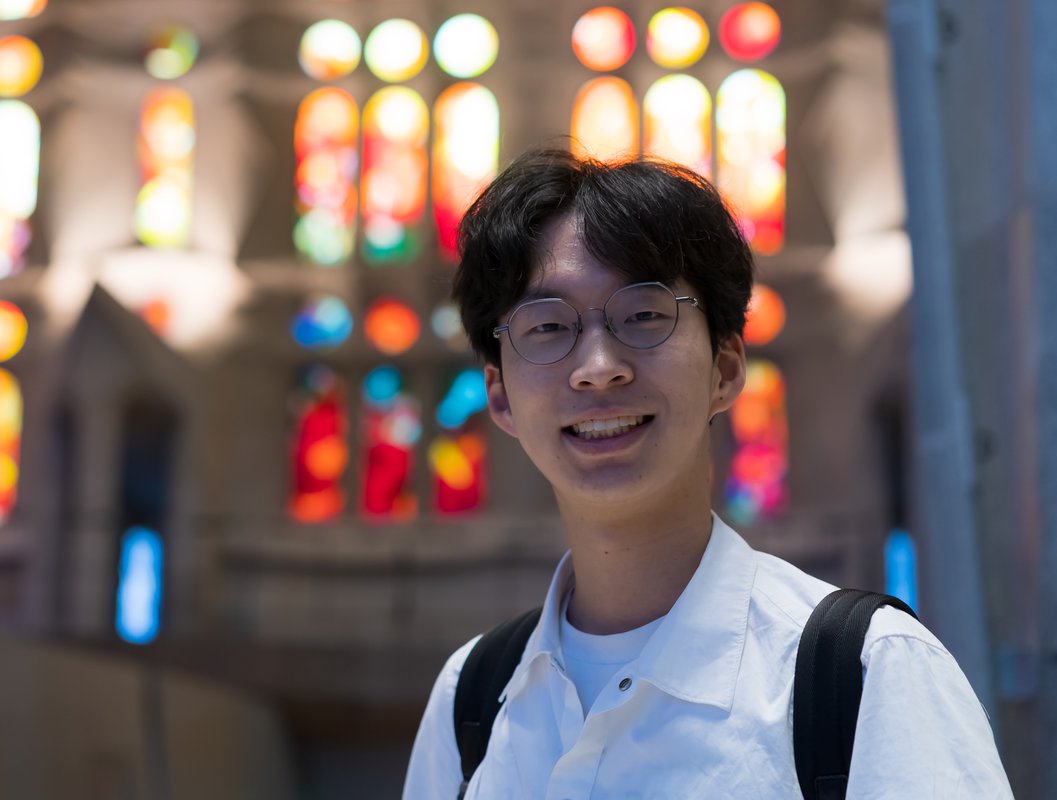
Anastasia Buyalskaya
Advisor: Colin Camerer // Social and Decision Neuroscience // G4
As an undergraduate, Anastasia majored in economics and worked in finance, drawn to markets which were presumed to be efficient and governed by rational individuals. But it became increasingly clear that there was a disconnect between what she was studying and seeing in the real world. "Investment experts were making decisions that were systematically influenced by their emotions, their cognitive biases, and their ego." At BlackRock, where she worked for five years before coming to Caltech, she worked with investment teams to understand and mitigate behaviors that were leading to suboptimal decision making, eventually launching a behavioral finance initiative and constructing a Behavioral Finance course for thousands of investors. "My work at BlackRock involved applying other people's research and I got the itch to do my own. I wanted to learn how to test theory, run experiments, and to answer questions about human behavior which hadn't been answered yet." Having seen how disciplines outside economics can shed light on economic models, Anastasia sought out a graduate school program that would be interdisciplinary (her field – behavioral science – is relatively new and came out of interdisciplinary collaborations), which led her to Caltech.
Anastasia's research focus has been understanding habit formation. "So much of our behavior is not a utility maximization problem. Think of brushing your teeth – most of us execute this behavior multiple times a day but give it very little cognitive attention. It is something we do automatically when we are cued by context." Anastasia explains that policies which aim to change behavior do not always work partly because those interventions appeal to the reflective, thinking mind rather than the automatic, habitual mind. She hopes her research – in partnership with her advisor Colin Camerer, and collaborators at the University of Pennsylvania – can shed light on how we might be able to better understand habitual behavior at the individual level and construct more targeted behavioral interventions. In addition to empirical work, Anastasia is interested in running experiments to study what leads habits to form. "It's very difficult to study habit inside the lab because by definition subjects are in a new setting and less likely to act automatically," she explains. So Anastasia set up a field experiment in which she would have the control of a lab but the context of the field: installing a customizable vending machine that would allow her to study natural choices on Caltech's campus – collecting data around the clock, when people desired one of the options available. While the COVID-19 pandemic put a halt on her experiments in the short-term, she's looking forward to the day she can resume studying human habits "in the wild."
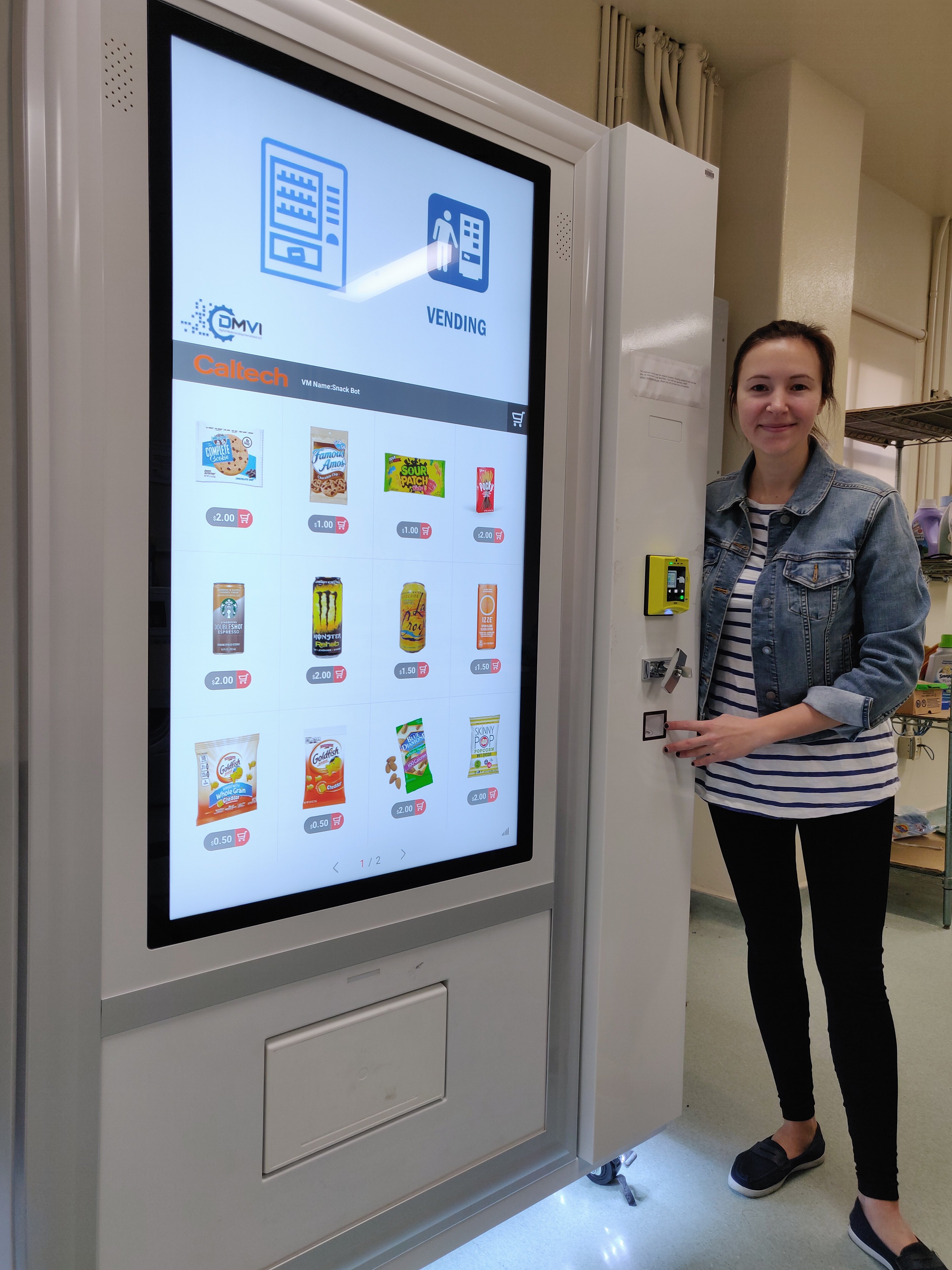
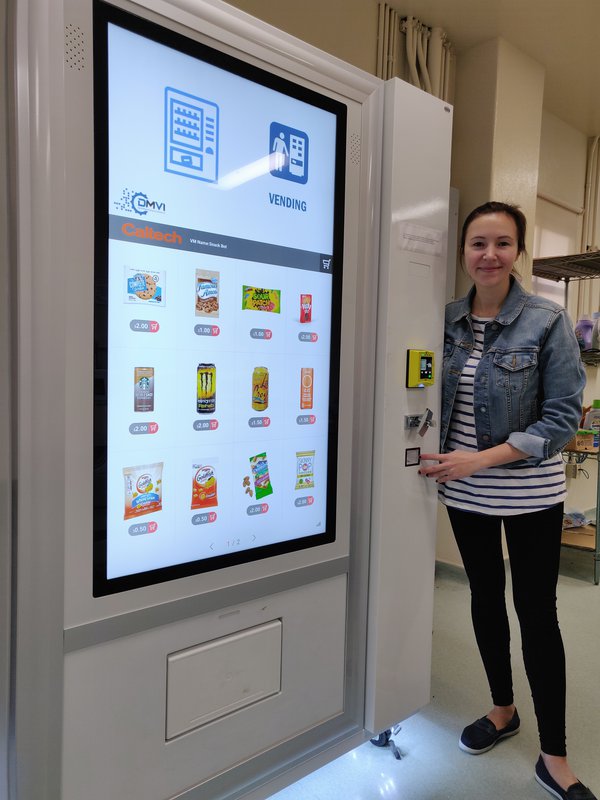